My research focuses on two major areas: 1) developing statistical and machine learning methods for the analysis of high-throughput biological data and implementing them in open-source software, and 2) applying such methods to analyse and interpret complex biological data to answer experimentally-driven questions.
I have contributed to popular methods for the analysis of differential expression in RNA-seq data, and recently have developed methods for the analysis of single-cell RNA-seq data. I primarily implement methods in the R and Python languages and publish open-source software packages through the Bioconductor project.
I am also interested in studying the effects of DNA variation on gene expression measured in individual cells. We can explore single-cell genetics in two ways: by studying effects of common DNA variation on single-cell gene expression (single-cell quantitative trait locus mapping) and by studying the effects of somatic DNA mutations on single-cell gene expression (clonal cell populations). The former provides information about genetic regulation of natural gene expression variation, while the latter informs us about the effects of DNA accumulated mutations in tissues that are relevant both to healthy ageing and to cancer.
I enjoy collaborating with biologists and other researchers to contribute computational and data analysis expertise to biologically-focused studies.
Key achievements
2021-2025 NHMRC Investigator Grant (Emerging Leadership 2)
2016-2020 NHMRC Early Career (CJ Martin) Fellowship
2011-2014 General Sir John Monash Scholarship
Related news
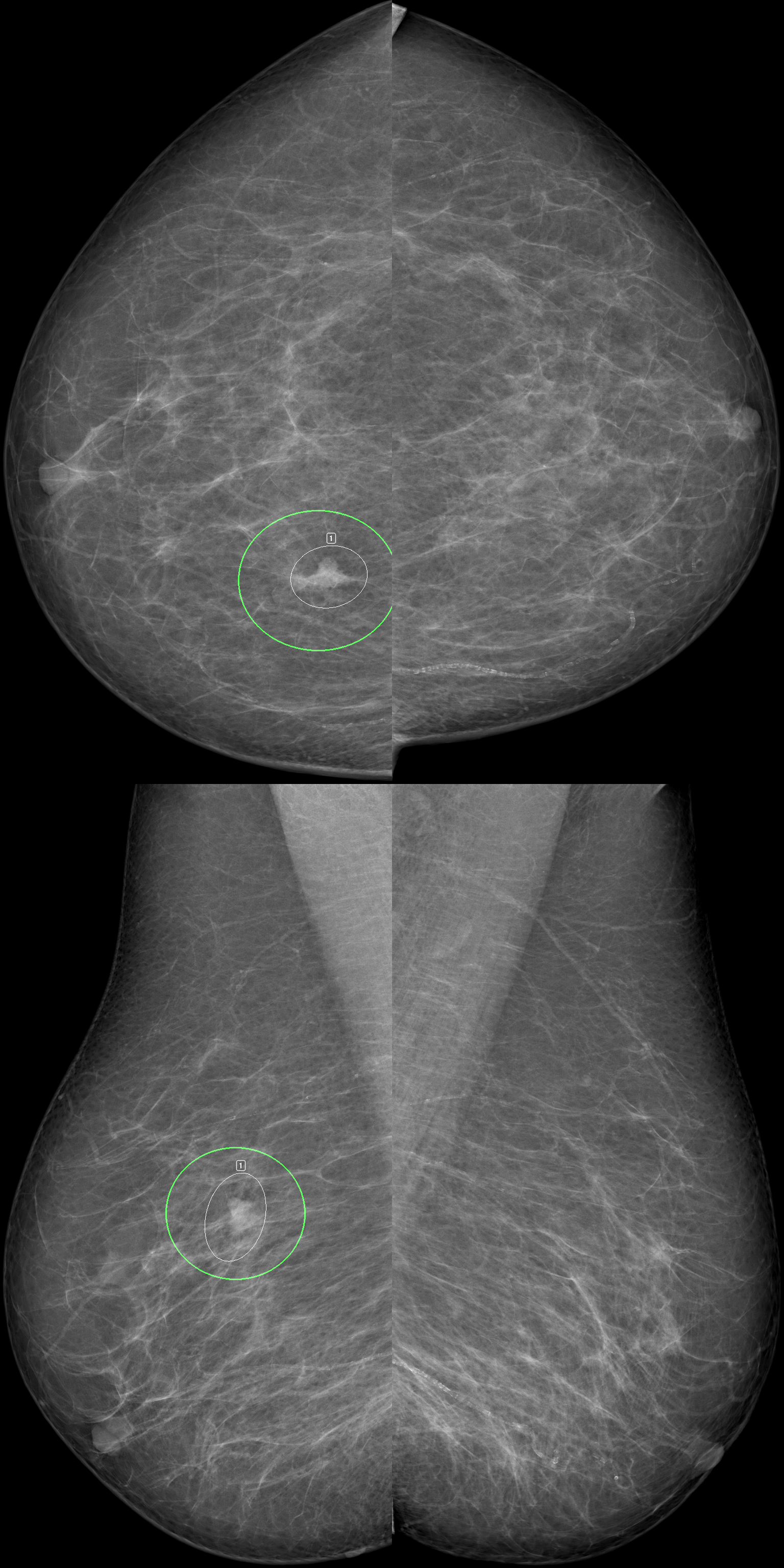
October 2024
AI helps boost breast screening accuracy
Research shows the benefits in integrating AI to read mammograms
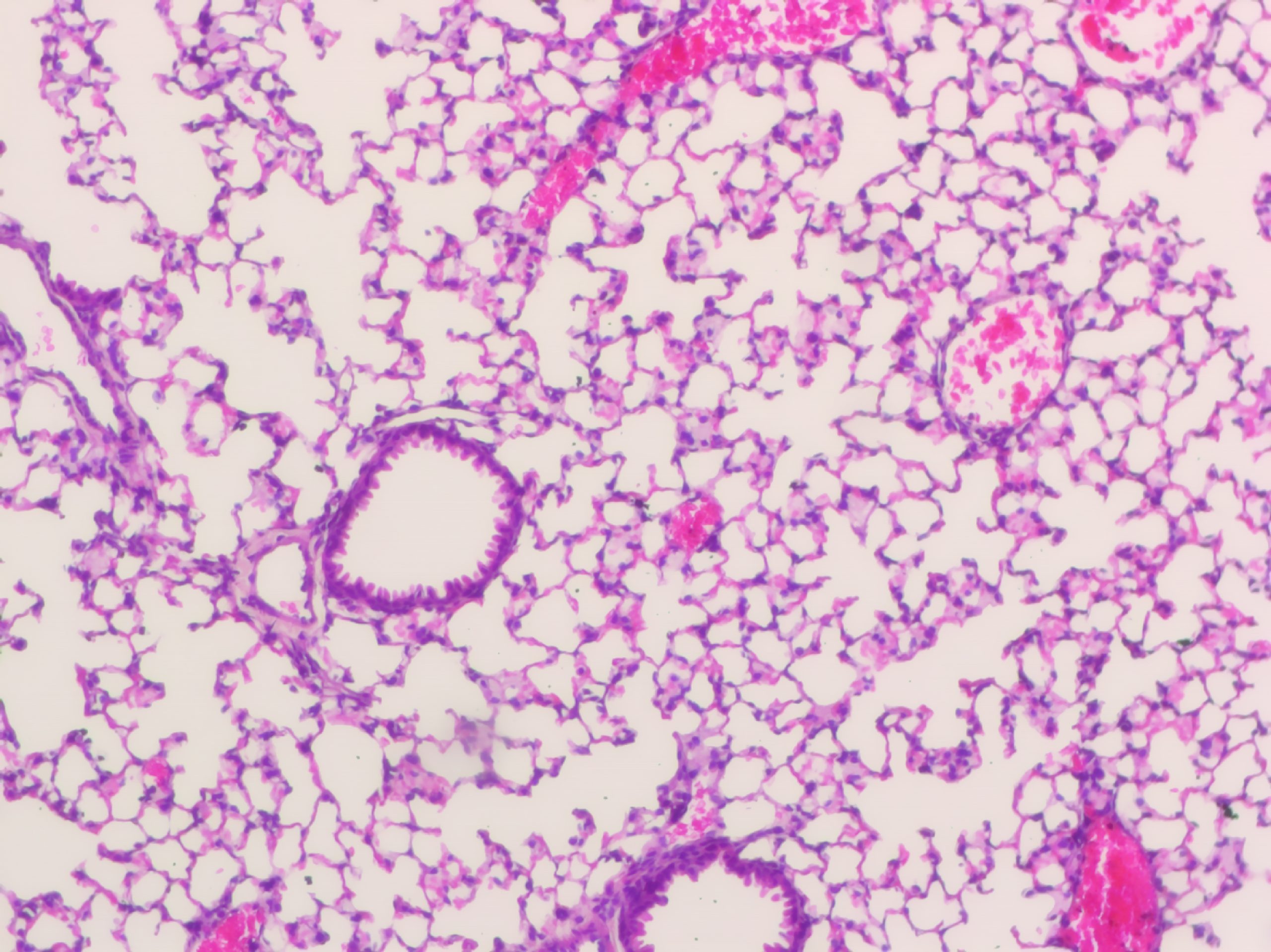
April 2024
Mapping the maze – New research sheds light on Idiopathic Pulmonary Fibrosis
Dr Davis McCarthy and collaborators have published a pioneering study on the genetic factors that influence the development and progression of Idiopathic Pulmonary Fibrosis (IPF), an incurable respiratory disease diagnosed in over 1,250 Australians each year.
Bioinformatics & Cellular Genomics
We focus on the challenges of analysing and interpreting large-scale biological data. Bringing together expertise in bioinformatics, statistics and machine learning, we develop new methods and software and collaborate closely with a wide range of colleagues on studies motivated by specific biologically-focused questions.
Lab head: Associate Professor Davis McCarthySelected publications
Natri, H. M., Del Azodi, C. B., Peter, L., Taylor, C. J., Chugh, S., Kendle, R., Chung, M.-I., Flaherty, D. K., Matlock, B. K., Calvi, C. L., Blackwell, T. S., Ware, L. B., Bacchetta, M., Walia, R., Shaver, C. M., Kropski, J. A.^, McCarthy, D. J.^, & Banovich, N. E.^ (2024). Cell-type-specific and disease-associated expression quantitative trait loci in the human lung. Nature Genetics. doi.org/10.1038/s41588-024-01702-0
Lyu, R., Tsui, V., Crismani, W., Liu, R., Shim, H., & McCarthy, D. J. (2022). sgcocaller and comapr: personalised haplotype assembly and comparative crossover map analysis using single-gamete sequencing data. Nucleic Acids Research. doi.org/10.1093/nar/gkac764
Azodi, C. B., Zappia, L., Oshlack, A., & McCarthy, D. J. (2021). splatPop: simulating population scale single-cell RNA sequencing data. Genome Biology, 22(1), 341. doi.org/10.1186/s13059-021-02546-1
McCarthy, D. J., Rostom, R., Huang, Y., Kunz, D. J., Danecek, P., Bonder, M. J., Hagai, T., Lyu, R., HipSci Consortium, Wang, W., Gaffney, D. J., Simons, B. D., Stegle, O., & Teichmann, S. A. (2020). Cardelino: computational integration of somatic clonal substructure and single-cell transcriptomes. Nature Methods, 17(4), 414–421. doi.org/10.1038/s41592-020-0766-3
McCarthy, D. J., Campbell, K. R., Lun, A. T. L., & Wills, Q. F. (2017). Scater: pre-processing, quality control, normalization and visualization of single-cell RNA-seq data in R. Bioinformatics, 33(8), 1179–1186. doi.org/10.1093/bioinformatics/btw777
McCarthy, D. J., Chen, Y., & Smyth, G. K. (2012). Differential expression analysis of multifactor RNA-Seq experiments with respect to biological variation. Nucleic Acids Research, 40(10), 4288–4297. doi.org/10.1093/nar/gks042
Robinson, M. D., McCarthy, D. J., & Smyth, G. K. (2010). edgeR: a Bioconductor package for differential expression analysis of digital gene expression data. Bioinformatics , 26(1), 139–140. doi.org/10.1093/bioinformatics/btp616
ORCID profile: https://orcid.org/0000-0002-2218-6833
Google Scholar profile: https://scholar.google.com/citations?user=A1F5_UEAAAAJ&hl=en